TRANSENSE
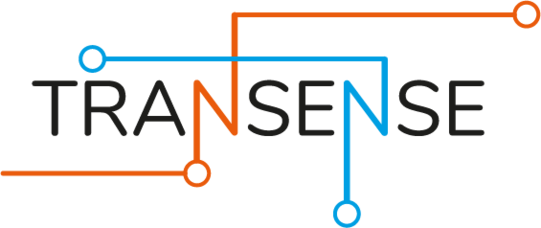
With the expansion of renewable energies, more grid transparency is necessary in order to continue to guarantee a stable grid operation. In transmission grids, state estimation has been successfully used to estimate the grid state based on available measurement data. However, distribution grids are not completely permeated with sensor technology, primarily due to historical and cost reasons. Installing sensor technology at each node to be observed is economically not feasible, which makes it difficult to impossible to transfer conventional state estimation technologies to the distribution grids. Therefore, an intelligent solution approach to create transparency is needed
In recent years the application of artificial neural networks to perform distribution system state estimation and thereby establish grid transparency, a combination called Neural State Estimation, has proofed promising. Unfortunately, a trained neural network is only applicable to the grid structure it is trained on. This is where TRANSENSE comes in: The goal of the project is to explore a transferable state estimation, for which transfer learning is applied to the Neural State Estimation. On top of that an innovative training method will be leveraged: If a model of the system is available - e.g., as a digital twin in operation - then the training algorithm monitors available training data und requests additional data from the simulator. These requests are based on a statistical analysis and determined to facilitate optimal training. The translation of statistical parameters into the parameterization of a simulation run is performed by a Simulation as a Service interface (SIMaaS).
Project Partners
Institut für Energiesysteme, Energieeffizienz und Energiewirtschaft (ie3), TU Dortmund Prof. Dr.-Ing. Christian Rehtanz | OFFIS e.V. – Institut für Informatik, Prof. Dr. Sebastian Lehnhoff | PSI Software AG, Dr.-Ing. Andreas Kubis | Netze BW GmbH, Dr.-Ing. Bartholomäus Wasowicz
Sponsors
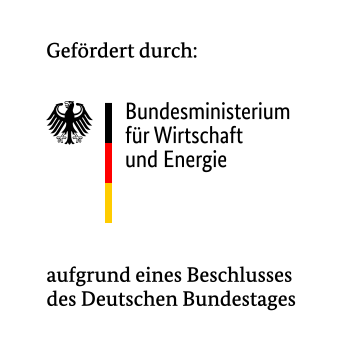
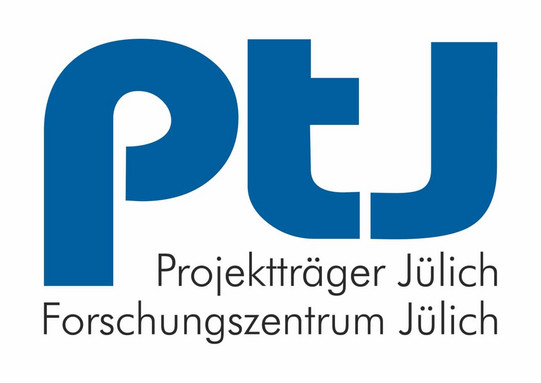