Transregio TRR391
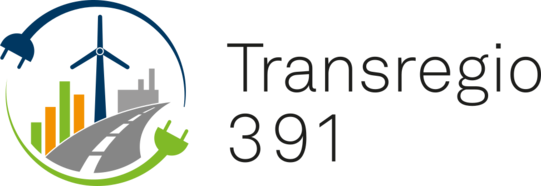
Spatio-temporal Statistics for the Transition of Energy and Transport
In TRR 391, we model, estimate, and predict spatio-temporal processes occurring in economic and technical applications. In doing so, we exploit the formal similarity of relevant statistical problems for methodological synergies and develop key techniques for the analysis of spatio-temporal data, which will enable efficient data-based decision-making in various areas of implementing the energy and transport transition over the next decades. ie3 is involved in the work package B01, which deals with statistical modeling and analysis for state estimation in electrical power distribution grids.
Classical state estimation techniques currently in use estimate the state variables at a single point in time. This is achieved by utilizing a sufficiently large number of measurements of spatially distributed sensors, which are time-synchronized within certain limits, and assuming a steady-state behavior of the measured values in a specific time interval of several seconds. However, in distribution grids, the number of spatially distributed sensors is significantly lower in comparison to transmission grids, where state estimation are ubiquitous in use. The measurements on distribution level have typically a lower temporal resolution and the steady-state assumption significantly reduces accuracy due to the high volatility of loads and feed-ins. Additionally, due to the limited number of measurements at sensors, less accurate pseudo-measurement values must be incorporated to achieve observability. Such pseudo-measurements are values derived from secondary data replacing a missing measurement. They are for instance obtained from weather reports to get the electrical input of wind power plants or photovoltaic systems, which might not be measured directly.
The first objective of this project is to design new methods which incorporate the temporal evolution of measured values and the formation of pseudo-measured values, as well as the spatial distribution of measurements, including correlations, into the estimation process. We will achieve this goal by determining important input variables, employing realistic model assumptions, and developing appropriate filtering methods. Specifically, we will relax the common normality assumption, which is unrealistic for electrical power distribution grids. This will lead us to new statistical approaches for the nonlinear state space models typically used in distribution grids, enabling robust estimation under highly volatile conditions.
Furthermore, on the distribution grid level, we need to ensure that voltage or power flows are kept within defined values with a specific confidence level for specific future time intervals. Therefore, our second goal is to determine the prediction intervals (confidence intervals) for the predicted (estimated) future values over a short time interval (e.g., 15 minutes) and to develop online change-point methods for detecting critical situations where the voltage or power flow will exceed critical values in the upcoming time interval. Expanding this time interval from short-term to daily operation is the long-term goal of the project.
To guarantee the required accuracy and probability, an optimal mix of sensors and different types of pseudo-measurements needs to be determined, and we will identify the minimum number of sensor measurements and their placement in medium and low-voltage networks. Additionally, we will determine optimal estimation intervals for the required accuracy. In a further step, the topology of the underlying grid model will be validated. Changes in the grid topology lead to modeling errors, which provide significant practical problems in today's analysis of distribution grids. To address these issues, we will develop new online change-point methods for detecting changes in the network topology that were not communicated to the network operator in a timely manner.
Project duration: 10/2024 - 06/2028
Project website: https://trr391.tu-dortmund.de/
Project partners
Technical University Dortmund
Fachgebiet Ökonometrie, JProf. Dr. A. Arsova | Arbeitsgruppe Computational Statistics, Prof. Dr. P.-C. Bürkner | Institut für Transportlogisitik, Prof. Dr. U. Clausen | Lehrstuhl für Ökonometrie und Statistik, Prof. Dr. Dr. M. Demetrescu | Lehrstuhl für mathematische Statistik und naturwissenschaftliche Anwendungen, Prof. Dr. R. Fried | Fachgebiet Statistical Methods for Big Data, Prof. Dr. A. Groll | Lehrstuhl für Wirtschafts- und Sozialstatistik, Prof. Dr. C. Jentsch | Chair of Uncertainty Quantification and Statistical Learning, Prof. Dr. N. Klein | Professur Applied Economics, Prof. Dr. L. Linnemann | Lehrstuhl Statistik mit Anwendungen im Bereich der Ingenieurwissenschaften, Prof. Dr. C. Müller | Institut für Energiesysteme, Energieeffizienz und Energiewirtschaft, Prof. Dr. C. Rehtanz | Fachgebiet für Mathematische Statistik, Prof. Dr. K. Schorning
Ruhr University Bochum
Chair of Mathematical Statistics, Prof. Dr. A. Bücher | Chair of Stochastics, Prof. Dr. H. Dette | Chair of Machine Learning, Prof. Dr. A. Fischer | Chair of Statistics and Econometrics, Prof. Dr. V. Golosnoy | Chair of Environmental/Resource Economics and Sustainability, Prof. Dr. A. Löschel
University of Applied Sciences and Arts Dortmund
Faculty of Computer Science - Mathematical Statistics, Prof. Dr. S. Kuhnt
Karlsruhe Institute of Technology
Chair of Data Science in Mechanical Engineering, Prof. Dr. A. Meyer
University of Duisburg-Essen
Chair of Econometrics, Prof. Dr. C. Hanck | Chair of Environmental Economics, esp. Economics of Renewable Energy, JProf. Dr. F. Ziel
University of Hamburg
Chair of Mathematics of Data-Driven Methods, Prof. Dr. J. Lederer
Hamburg University of Technology
Institute of Control Systems, Prof. Dr. T. Faulwasser
University of Münster
Chair of Remote Sensing and Spatial Modelling, Prof. Dr. H. Meyer | Institute for Geoinformatics, Prof. Dr. E. Pebesma
RWI Leibniz Institute for Economic Research (RWI)
Department of Environment and Resources, Prof. Dr. M. Andor, Prof. M. Frondel, Prof. Ph.D. C. Vance | Research Data Center Ruhr, Dr. P. Breidenbach
Sponsor
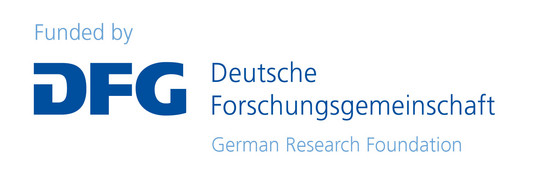